Cross post – https://www.linkedin.com/pulse/introduction-text-chunking-purposes-vector-embedding-arumilli-zf6vc
In a previous post “An introduction to vector embeddings”, I have mentioned about vector embeddings and have posted a link for MTEB leaderboard. In this article I am going to discuss about “chunking”.
WebVeta does this and much much more in the A.I tier, you can sign-up, add your domains, blogs, validate ownership of your websites, few settings, copy and paste 2 – 3 lines of HTML and relax. WebVeta has a KickStarter campaign running and offering significant discounts via KickStarter.
If you see in the MTEB leaderboard, the 6th column Max tokens. Max tokens is the number of tokens accepted by the embedding model.
When using embedding models that have smaller max tokens and when we want to store large texts, we need to chunk the text.
In certain cases, even if a embedding model uses a large Max tokens, we want to look for specific information in a text document, even then we need to chunk. Let’s say I have a document that has 30,000 chunks, when I am searching for something, if I need entire document then I can use model with 32k max tokens. But what if I want just a small paragraph that has the information and if I don’t care about the rest of the document? For handling this looking for specific information type of scenario, we can chunk the documents into smaller parts and then index the chunks rather than the whole document.
For example: “Mr. Kanti Kalyan Arumilli is the founder and CEO of ALight Technology And Services Limited and ALight Technologies USA Inc” has approximately 33 chunks.
There could be other use-cases for chunking.
But chunking loses the context. In a large document, let’s say about some person, when the entire document is in context, we know from the entire text that the document is about the person. When we use chunks, some paragraph might use the term ‘he’ / ‘him’, and when the paragraph is seen in isolation, we lose the context. Too small chunks are a problem, too big chunks are not useful in certain use-cases.
Various approaches need to be considered for retaining context or adding context. There are various approaches and there is no absolute answer of what’s the best chunk size. I have seen decent results for chunks of sizes between 384 – 1024 tokens.
I am planning few more blog posts over the next few weeks regarding LLM’s RAG’s, Vector Databases, Storing, Retrieving etc…
–
Mr. Kanti Kalyan Arumilli
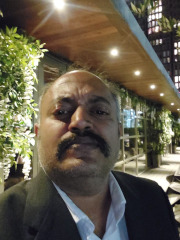
B.Tech, M.B.A
Founder & CEO, Lead Full-Stack .Net developer
ALight Technology And Services Limited
Phone / SMS / WhatsApp on the following 3 numbers:
+91-789-362-6688, +1-480-347-6849, +44-07718-273-964
kantikalyan@gmail.com, kantikalyan@outlook.com, admin@alightservices.com, kantikalyan.arumilli@alightservices.com, KArumilli2020@student.hult.edu, KantiKArumilli@outlook.com and 3 more rarely used email addresses – hardly once or twice a year.